It’s been quite some years since AI has been trendy in the world of business, particularly for startups, and even more when talking to VCs. There have been hundreds of headline-grabbing discussions of futuristic applications of AI and an AI hype in the startup world as a result. They often depicted AI as the cornerstone of their competitive advantage, capacity for disruption of an already existing market, or the emergence of a totally new market. However, when investigating an AI startup for a funding round, it is often difficult to distinguish impactful AI solutions from ideas that only use AI as a buzzword or marketing catalyst. Some startups might use AI in their business, but figuring out the core impact and use of AI on the startup business model usually remains foggy without a deep dive into the company’s activities. As a VC, having the correct analytical elements when investigating AI startup investments becomes key if not essential.
Nevertheless, given the variety of AI applications we consider, whether AI is employed as a development facilitator, a data analytics provider, a deep tech researcher tool, or more directly AI-powered provider of goods and services, building a timeless framework on how to measure the impact of AI in a business may appear presumptuous. However, scientific literature gives us some interesting insights on elements to consider while evaluating the true impact of AI in companies while enlightening the key elements AI-powered startups need to scale and grow accordingly. We had the chance to work on the review of more than 100 academic references on AI startups, in the context of Maxime Lhoustau’s Master Thesis for HEC Paris and TU Munich. In this article, you will find the key takeaways, and the complete explanations of every point and the full text, as well as all references, will soon be available for those interested to dig into a specific point.
How do we evaluate the impact of AI on startups’ business models?
As quickly defined in the intro, the nature of an AI-powered startup can be classified into four categories: AI-charged product/service provider, AI development facilitator, data analytics provider, and deep tech researcher. However, the nature of these business models doesn’t say anything about the disruptive potential of these solutions. AI can indeed be implemented in different ways, that are not all equal to VCs in regards to the impact they have on the business itself.
Pfau and Rimp (2021) proposed to map AI solutions on a matrix according to their influence on the company’s business model and to infer corresponding strategic zones: “Outsourcing”, incremental or profound changes, and “Disruption”. This mapping should be done based on the impact – minor, moderate, and grand – the corresponding AI solution has on the company’s own activities (the way the company carries out activities, such as cost structures of partnerships), and on its customer-specific activities (activities related to the customer, such as communication or channels).
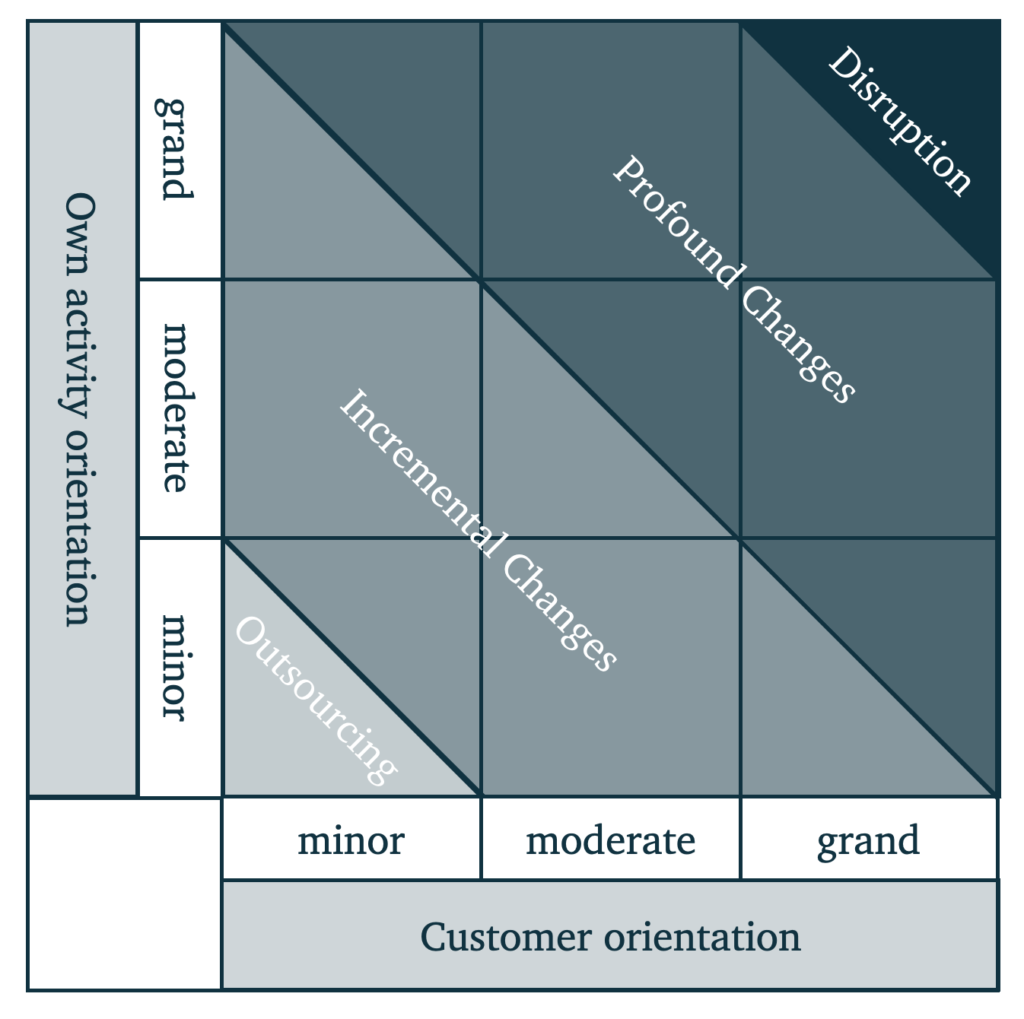
The first step in assessing the impact of an AI initiative on a business model is then to evaluate the intensity it has on the company’s and its clients’ activities, and then map the initiative on the matrix. The second step is to infer the corresponding strategic zone on the matrix. If the mapped initiative falls into the “Outsourcing” or “Incremental Changes” zones, it shows that this project has a small to moderate impact on the company’s business model, which may choose to outsource the development or consider more impactful applications. On the contrary, if an AI initiative falls into the “Profound Changes” or “Disruption” zones, its impact on the company’s business model is significant. Overall to be considered AI-powered startups in our eyes, AI must be a critical component that not only allows for business model innovation but also makes this specific business model possible. VCs are particularly interested in this kind of AI initiative because it can result in a strong competitive advantage, the disruption of an already existing market, or the emergence of a totally new market.
How do we analyze the organizational structure as well as the culture and talent management of an AI-powered startup?
Scholars agree that AI technologies require a complete new flat, agile organization with an AI factory at its core to best leverage AI capabilities. The AI factory is generally made of four components: the data pipeline, the algorithms, an experimentation platform and the IT infrastructure. To achieve such flexibility and AI drivability, human and AI resources must be integrated vertically and horizontally from product creation to strategic decision-making. Leaders should form interdisciplinary and cross-functional teams that have the power to conduct initiatives, while a new test-and-learn mentality approach should be recommended to these teams to embrace risk and become experimental and adaptable. For example, some startups have been able to encourage the spontaneous formation of teams when a project is initiated, which then dissolves when the work is done. Such flexibility in resource allocation to projects is key to the development of AI initiatives.
We may therefore focus on investigating the level of agility of the potential candidates for funding, as the more flexible AI startups are, the more AI technologies can be leveraged. Moreover, we might also investigate the composition of internal teams and insist on the importance of interdisciplinary collaboration for good AI performance.
The use of AI technologies comes with specific risks that can be reduced through the continuous assessment of the startup’s AI readiness to pilot its activities. To do so, Jöhnk et al (2021) propose a broad list of AI readiness factors with corresponding AI characteristics and organizational necessities (cf figure below) that can help startups prepare for piloting their AI activities, in areas such as strategic alignment, resources, knowledge, culture, and data. To limit the potential failures of AI and to build trust among customers, AI startups may adopt a risk management program with appropriate risk communication in case of emergency.
We might use some elements of the AI readiness factors for a first evaluation of the AI startups’ capacity to undertake AI, such as AI-business potentials, customer AI readiness, IT infrastructure, innovativeness, or even AI ethics and data availability. We may also raise awareness among AI startups in our portfolio about specific AI readiness factors that might not be the first priority in the early ages, but that could become an obstacle for future growth such as data-driven decision making, personnel upskilling, or specific financial budget for AI adoption. Another way to reduce global uncertainty is to bring portfolio startups’ attention to risks that accompany AI adoption and to encourage them to undertake risk management and communication initiatives to temper potential emergencies.
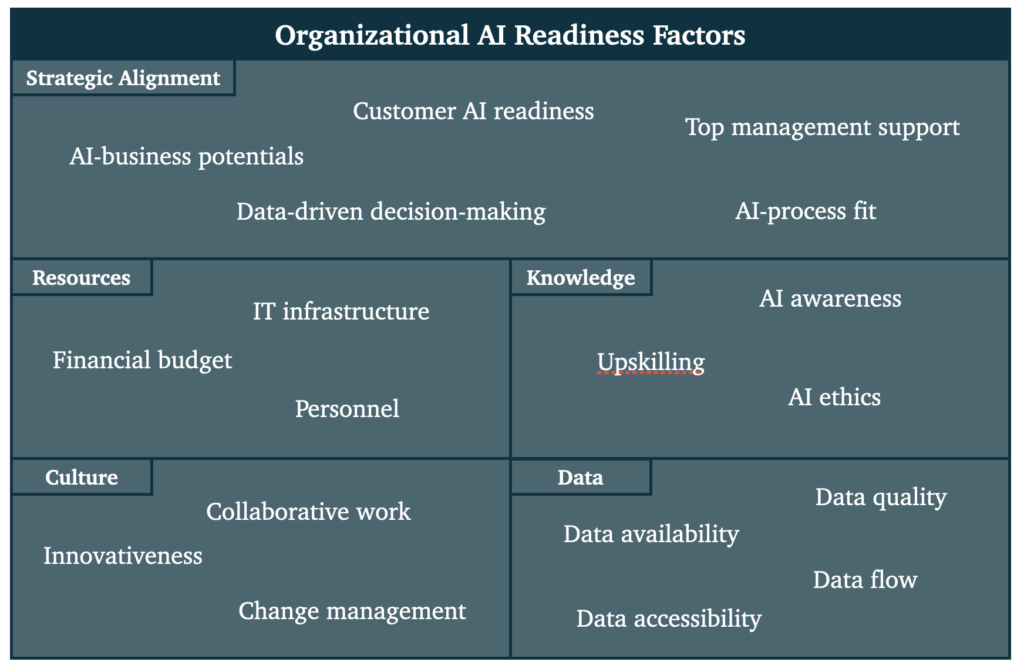
Talent is the scarcest resource along with data when it comes to AI, with the most important skills to demonstrate being evaluating and training AI algorithms from scratch as well as deploying them. Thus, specific continuous training and AI education are strongly recommended at all levels, from end-users to analytics and leaders. To support the new test-and-learn mentality, managers may internally show examples of successful AI projects and insist on the lessons learned from failures. Moreover, the use of widely shared OKRs instead of KPIs seems to be preferred to show the path to success and develop transparency in the organization. Building an AI-friendly company culture is also a determining factor to establish successful AI applications, and may be engaged by encouraging innovativeness and collaborative work within the organization.
VCs could therefore investigate what the talent strategy of AI startups is, how they are building a favorable company culture for AI, and what specific metrics they are monitoring. In addition, they may help recruit the right talents for their portfolio’s AI startups by understanding what type of profiles and skills are the most needed, and by encouraging startups to implement specific retaining programs for key talents.
How do we evaluate data accessibility issues for AI-powered startups, and how to handle the corresponding risks and regulations with ethical AI?
Data accessibility plays a crucial role in AI applications, being the scarcest resource with talents for AI startups. Data, and especially proprietary data, play the role of strategic defensible barriers for businesses as well as a competitive advantage, enabling the development of more valuable products. Accessing data is then crucial, but not enough as data must also be qualitative to enable the development of performing AI models. However qualitative data is difficult to define as there is no “one-size-fits-all” approach to the different existing AI technologies, but qualitative data is globally recent, diverse, and accessible for the startup. Startups should consider any data as a potential candidate for AI to be able to seize future opportunities. Moreover, data accessibility is a common issue for AI startups and should be managed as a business issue first and through early-on discussions at the onset of AI initiatives.
Data network effect is an interesting mechanism to be constantly researched by AI startups. A platform exhibits data network effect if, the more the platform learns from the data it collects on users, the more valuable the platform becomes to each user. Such an effect results in a more personalized and meaningful experience for users while reinforcing the competitiveness of the firm and giving rise to the “winner-takes-all” effect. To access data and specific expertise, AI startups may also partner with large high-tech firms and form precious partnerships.
At Alven, understood that data is what fuels AI applications, and therefore it should be treated with careful attention. As a result, we are always looking for startups that have access to a sustainable proprietary data source, as this in itself is a competitive advantage in the AI sector. We may also ask startups where they are sourcing data and if there is a risk that this data source can dry up. In addition, we might support AI startups regarding data by encouraging partnerships between startups in our portfolio, or by leveraging our network to put AI startups in touch with big high-tech firms.
When it comes to handling data (and especially personal data), regulations and risks are to be taken into account. The principal concerns arise when AI startups deal with the monitoring of individuals and respect for privacy. Algorithmic bias, unrepresentative sample of data, or wrong construction of algorithms can indeed be responsible for huge damages. The corresponding challenges AI startups face when dealing with these issues are the implementation of specific governance, how to manage internal compliance and potential brand damage in case of mistakes. Of course, GDPR is supposed to help startups define the borders of what they are allowed to do, and often leads to the creation of new positions in firms to handle regulatory issues. Moreover, the adoption of ethical AI principles seems to be a key asset for AI startups, as it helps respect GDPR and signals willingness to adopt the industry’s norms, resulting in easier collaboration with other firms and investors.
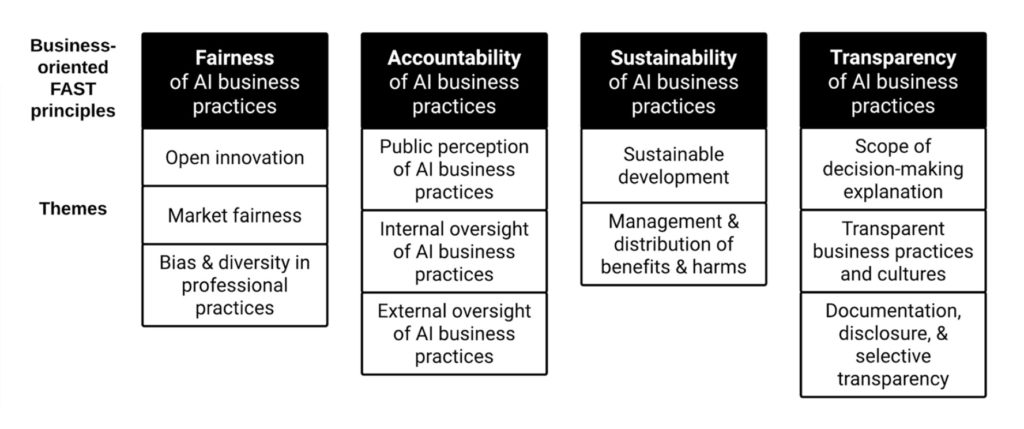
There is nevertheless no agreement upon a single set of ethical AI practices to undertake for AI startups, but the FAST principles are a good start for startups: fairness, accountability, sustainability, and transparency (cf figure above). Fairness necessitates that AI developers and users do not harm others as a result of potentially biased or discriminating outcomes of AI business practices. Accountability mandates that businesses provide justification for entrusting AI-powered business decisions to human creators and operators, and that there be adequate safeguards in place to keep those enterprises accountable for their choices. Sustainability requires leveraging AI business practices to achieve positive transformative and long-term effects on individuals and society. Lastly, transparency necessitates the capacity for the public or government to comprehend how and why AI operated as it did in a certain business context, and thus the rationale behind its choice or behavior. For AI startups, understanding potential biases in data and algorithms, implementing checks, better educating technical workers, increasing diversity and making ethical AI a global issue within the organization are ways to start implementing ethical AI principles. Influencers such as large AI companies or public institutions share their own set of ethical AI guidelines, but as there is yet no consensus in the AI community about a correct single set of principles, they have to be carefully considered.
VCs may thus start investigating what measures the considered AI startups are undertaking to be RGPD compliant, and how they internally handle sensitive private data. Ideally, we would try to measure the potential risks associated with the presence of biases, misuse, or misappropriation of the AI startups’ products. Once in the portfolio, we may support AI startups by sensitizing them to the risks of using AI models and by sharing the beneficial sides of adopting ethical AI principles. Insisting on adopting ethical AI principles would help startups to reduce risks and uncertainty while reassuring investors about their capacity to adapt to the industry’s norms and be better candidates for future exits.
How do we assess AI-powered startups’ capacity to grow and scale?
AI startups’ growth is a double-edged sword. On the one hand, AI technologies can activate a double virtuous growth cycle to enhance the scale and the scope of the company thanks to data network effects. AI-powered startups can even extend the concept of scaling by being able to transfer mature AI models to other business use cases. On the other hand, several factors can slow down the scaling process of AI-powered startups. Indeed, expensive cloud infrastructure for the training of AI models as well as the long AI models development time may cause technical slowdowns. Moreover, we see lower and lower entry barriers in the AI industry with the democratization of open source, no-code or developer tools that ease the painful development process of AI models. As we previously mentioned, data is crucial for AI startups and may even become a bottleneck for growth at some point. Therefore, AI startups have a higher need for investing in securing data and human resources.
VCs are particularly interested in hypergrowth, and thus we are constantly looking for companies that can successfully leverage data network effect to fuel their growth. The transfer of mature AI models to other verticals is also of interest to us, and we may try to anticipate what other markets AI startups could enter when being more mature.
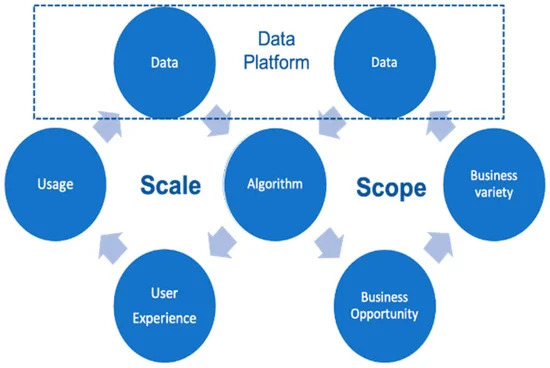
Successful AI startups usually scale by utilizing a different operating model, organized around an AI factory. The restructuration of the firm goes with a strategy, a clear architecture, an agile product focus, and multidisciplinary governance. At this point, the implementation of an ethical AI governance that supervises and manages AI initiatives as well as the associated risks seems to be mandatory not to lose control over a big number of deployed AI models. Moreover, an intellectual property strategy and a proper budget for AI integration and adoption might be adopted. AI startups in the process of scaling up may develop a portfolio of AI initiatives and prioritize them based on a long-term view (typically 3 years) and take into consideration potential synergies between them to maximize value. Lastly, mature AI startups might engage in young AI startups investments to stay at the edge of the technology, enter new markets, and build a precious ecosystem to strengthen their leadership position.
When a startup of our portfolio starts to sustainably grow and scale accordingly, we can especially help them manage the political risks associated with AI in addition to their usual support. Indeed, political risks are the most significant threats that businesses face when expanding globally, and we can assist AI startups in developing solid governance mechanisms to address various legal and ethical issues associated with AI and big data.
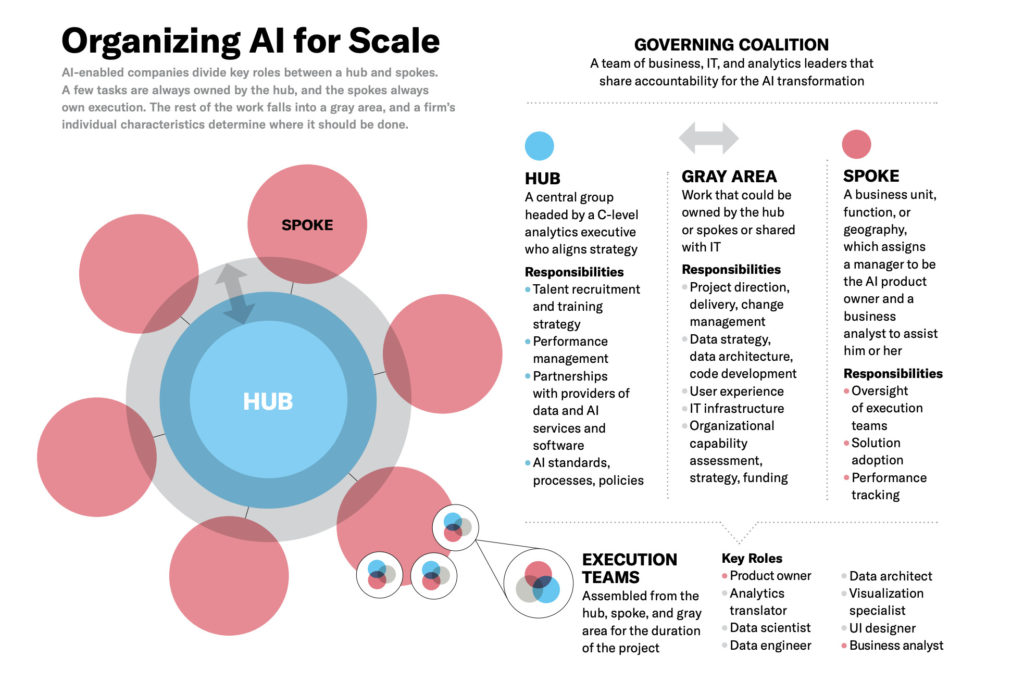
With all these in mind, the following 10 questions should be asked by early-stage VCs assessing the potential impact of AI in startups :
- What is the impact of your AI projects on your business model?
- Are you able to form flexible teams, with technical, business and management skills within them? Can they take initiatives?
- Did you assess the risks associated with the use of AI models?
- How do you attract talent, and how do you retain them?
- What are your data sources, and are they sustainable?
- How do you deal with GDPR and sensitive data?
- Are you following any ethical AI guidance?
- Can you leverage data network effects and AI models synergies to accelerate your growth?
- Do you consider creating ethical governance to secure and plan AI projects?
- Are you building an organizational structure that will be able to support your growth and make your company digitally operated?
For those interested in going deeper into the AI sector, we recommend the following academic papers to read :
- Organizational structure:
- Building the AI-powered Organization in the Harvard Business Review, by Tim Fountaine et al (2019)
- Ready or not, AI comes—an interview study of organizational AI readiness factors in Business & Information Systems Engineering 2021 Vol. 63 Issue 1, by J. Jöhnk et al (2021)
- Governance, risk, and artificial intelligence in AI Magazine 2020 Vol. 41 Issue 1, by A. Mannes (2020)
- People, company culture and management:
- The Secret to AI is People in the Harvard Business Review, by Nada R. Sanders and John D. Wood (2020)
- Factors inhibiting the adoption of artificial intelligence at organizational-level: A preliminary investigation in Twenty-fifth Americas Conference on Information Systems Cancun 2019 by S. A. Alsheibani et al (2019)
- Data and GDPR:
- The role of data for AI startup growth in Research Policy 2022 Vol. 51 Issue 5, by J. Bessen et al (2022)
- The role of artificial intelligence and data network effects for creating user value in Academy of Management Review 2021 Vol. 46 Issue 3, by R. W. Gregory et al (2021)
- Ethical AI:
- Beyond the promise: implementing ethical AI in AI and Ethics 2021 Vol.1 Issue 1, by R. Eitel-Porter (2021)
- The Ethics of AI Business Practices: A Review of 47 AI Ethics Guidelines in AI and Ethics, by B. Attard-Frost et al (2022)
- Business Models:
- AI-enhanced business models for digital entrepreneurship in Digital Entrepreneurship, W. Pfau and P. Rimpp (2021)
- AI Startup Business Models in Business & Information Systems Engineering 2022 Vol. 64 Issue 1, by M. Weber et al (2022)
- A business model template for AI solutions in Proceedings of the International Conference on Intelligent Science and Technology 2018 by I. Metelskaia et al (2018)
- Scaling and Growth:
- Competing in the Age of AI in the Harvard Business Review, by Marco Iansiti and Karim R. Lakhani (2020)
- A scaling perspective on AI startups in Proceedings of the 54th Hawaii International Conference on System Sciences 2021, by M. Schulte-Althoff et al (2021)
- Artificial Intelligence Factory, Data Risk, and VCs’ Mediation: The Case of ByteDance, an AI-Powered Startup in Journal of Risk and Financial Management 2021 Vol. 14 Issue 5, by P. Jia and C. Stan (2021)